Colossal-AI accelerates Protein Structure Prediction by up to 11 times
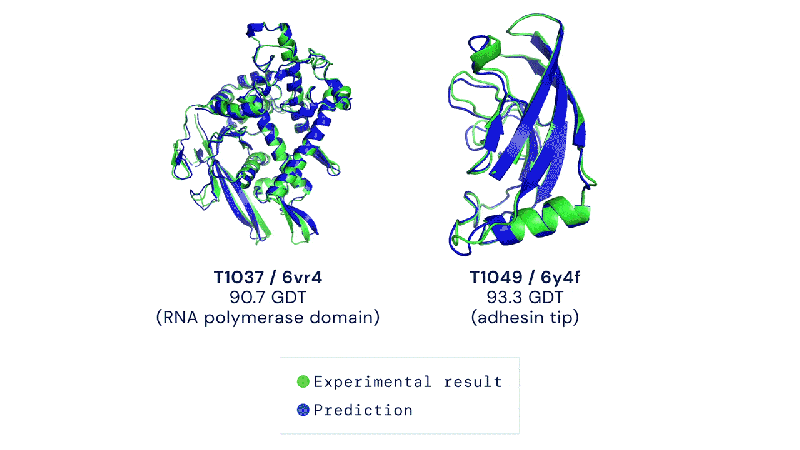

The Colossal-AI team and BioMap have created a new tool called xTrimo Multimer that can quickly predict the 3D shape of both single proteins (monomers) and multiple proteins that work together (multimers) using AlphaFold but optimized for a faster runtime.
Reduced training time
from 11 days to only 67 hours
Accelerated inference time
by up to ~11.6 times
"The collaboration with HPC-AI Tech brings together the cutting-edge technology in large AI model training from Colossal-AI and the biocomputing domain expertise from BioMap. The release of xTrimo Multimer is an important step towards integrating the advantages of large AI model training and inference into the construction of BioMap’s xTrimo multimodal system."
About the customer
BioMap is a team of world-renowned scientists who have extensive expertise in disease biology, bioinformatics, machine learning/deep learning, and antibody engineering. BioMap was co-founded by Baidu’s Founder/CEO Robin Li and the former CEO of Baidu Ventures, Wei Liu. They are committed to bringing first-in-class medicine for unmet medical needs in the areas of immune-oncology, autoimmune diseases, fibrosis and aging-related diseases.
-
Industry: Biotechnology
-
Solution: Performance optimization for deep learning to predict protein structures
-
Model: AlphaFold
-
Product: Colossal-AI FastFold
The challenge
Protein structure prediction is one of the most important topics in structural biology and supplements the understanding of gene translation and protein function. It is a process where scientists try to figure out the 3-dimensional shape of a protein from its genetic code (amino acid sequence). Knowing the structure of a protein is important because it can reveal how the protein works and how it interacts with other molecules. This information can be used to design new drugs, understand and treat diseases, and develop new technologies.
Unfortunately, the multi-level structure and sophisticated protein interactions make it challenging to predict the 3D structure accurately. In recent years, the success of deep neural networks has transformed various practices. Since DeepMind’s release of AlphaFold (which accurately predicts protein structure based on amino acid sequences), the field of biology has witnessed a boom in the utilization of AI for protein structure prediction.
Specifically, AlphaFold can generate end-to-end 3D structure predictions of protein monomers directly from amino acid sequences. The use of AlphaFold also exceeds the realm of monomers. Since the majority of proteins function as multimers, DeepMind’s AlphaFold-Multimer model is recently released to be able to predict the structure of multimers.
The solution
To boost the development of AlphaFold, the Colossal-AI team has already released FastFold, an open-source and optimized implementation of AlphaFold. The Colossal-AI team managed to successfully minimize AlphaFold’s training time from 11 days to only 67 hours and accelerate inference time by up to ~11.6 times. The Colossal-AI team continues its efforts to democratize large-scale AI model applications in the pharmaceutical field.
Interactions between proteins are critical to their biological functions. To address difficulties related to protein monomer and multimer structure prediction, the Colossal-AI Team proposed the industry’s latest solution, the xTrimo Multimer. xTrimo Multimer is able to better reflect protein interactions, thus enhancing the potential target analysis, protein structure prediction/ simulation, as well as high-precision antibody designs in drug discovery and development.
The unaffordable economic and time costs from AlphaFold’s inference has led to challenges in its research and development, particularly when facing long sequence inferences with rising computational complexity and memory consumption. Based upon computational features in the AlphaFold-Multimer model, the Colossal-AI team introduced CUDA optimization and Kernel Fusion techniques for the xTrimo Multimer achieving remarkable inference performances.
The results
Compared to AlphaFold2 and OpenFold (from Columbia University), the xTrimo Multimer has a significantly improved inference performance on a single GPU by 1.58-2.14 times and 1.14-2.23 times, respectively.
Additionally, the xTrimo Multimer model supports distributed inferences for lengthy sequences. After introducing Dynamic Axial Parallelism, the xTrimo Multimer was able to efficiently distribute computation and partial GPU memory across a variety of devices, thereby solving computational and memory challenges that long sequences face. xTrimo Multimer achieves a 8.47x and 11.15x speedup compared to OpenFold and AlphaFold 2 on multiple GPUs with sequence lengths ranging anywhere from 2-3K. xTrimo Multimer also shows a 4.45x acceleration compared to Uni-Fold 2.0. Furthermore, xTrimo Multimer can support inferences with sequences reaching up to 4K, whereas OpenFold and AlphaFold 2 are restricted from such lengths due to GPU memory. With xTrimo Multimer, scientists can run a 4K length sequence inference in about 20 minutes.
The future
"Our latest solution of protein Monomer and Multimer structure prediction is an important progress of Colossal-AI to solve the industrial problems in the real world. In the future, we will continue to cooperate with BioMap more deeply in biocomputing large models, to stimulate the application and implementation of deep learning in innovative drug development. "